How Data Management is Essential for AI Success
Artificial Intelligence (AI) is redefining the future of manufacturing, bringing unprecedented levels of efficiency, precision, and innovation to the industry. As manufacturers face increasing demands for higher quality, faster production times, and reduced costs, AI emerges as a game-changing tool that can meet these challenges head-on. From predictive maintenance that prevents costly downtime to intelligent automation that enhances production processes, AI is revolutionizing how products are designed, produced, and delivered.
Yet, behind the impressive capabilities of AI lies a fundamental truth: without data, AI cannot exist. The phrase "No Data, No AI" succinctly captures this reality, highlighting the critical role that data plays in powering AI systems. This fact is corroborated by the survey of 300 manufacturers across the world that have begun working with AI conducted by MIT Technology Review Insights in partnership with Microsoft. Most of the respondents (64%) are currently researching or experimenting with AI. About 35% of the respondents have begun to put AI use cases into production. Many executives that responded to the survey indicate they intend to increase AI spend significantly over the next two years. Those who haven’t started AI in production are moving gradually. To facilitate use-case development and scaling, these manufacturers must address challenges especially in the area of data.
Some of the key insights from the survey include:
Constraints on AI Scaling
Talent, skills, and data were identified as the main constraints on AI scaling in the areas of product engineering and design as well as factory operations. While talent and skills can be somewhat addressed through better hiring practices, training programs and awareness, data remains one of the toughest challenges in scaling AI use cases for manufacturers. The closer use cases get to production, the harder this deficit bites. Many respondents specifically highlighted inadequate data quality and governance as a major constraint. Insufficient access to cloud-based compute power that can hamper the data processing needed for AI is another oft-cited constraint in engineering and design.
Top Use Cases Identified for AI
Some of the top use cases for AI like quality management, maintenance in the pilot phases and conversational AI, product design and process optimization in production need good quality data for AI to be initially implemented and scaled.
Scaling can stall without the right data foundations. Respondents are clear that AI use-case development is hampered by inadequate data quality, weak data integration, and weak governance. Only about one in five manufacturers surveyed have production assets with data ready for use in existing AI models. That figure dwindles as manufacturers put use cases into production. The bigger the manufacturer, the greater the problem of unsuitable data is.
The Internet of Things (IoT) data management strategy could be an effective way to overcome some of the AI data challenges mentioned above. A data management strategy ensures that the data is effectively consolidated from all the data silos seen within the manufacturing operations, transformed to a common format, normalized and contextualized so that it is ready to be used for AI use cases. One of the ways to implement an effective data management strategy is by using an MQTT platform like HiveMQ, which offers a suite of solutions enabling an enterprise-grade data management solution.
One of the ways HiveMQ is able to support our customers on this journey is by implementing a data framework named Unified Namespace (UNS) (Fig. 1) which serves as the single source of truth for all contextualized data and information of the manufacturing business. At the core of UNS is the Enterprise data broker offering a highly scalable, reliable, and flexible data management solution.
Figure 1 – HiveMQ IIoT Enterprise Data Architecture for Smart Manufacturing
The other key aspect of this is the HiveMQ Data Hub (Fig. 2) which provides an integrated policy and data transformation engine that validates, enforces, and manipulates data in motion to ensure data integrity, quality, and governance across the deployment.
Figure 2 – HiveMQ Data Hub offering data policies and transformations
AI Spend and Value Gained
When looking at who spends the most on AI projects, it's clear that the biggest players with the most budgets do the most spending, and also have the highest expectations of the outcomes from AI. In engineering and design, most executives expect their organizations to increase AI spending during the next two years. Most say the same when it comes to factory operations. The largest manufacturers are far more likely to make big increases in investment than those that are relatively smaller. One of the reasons why bigger companies are able to spend big on AI is that they are better able to drive the other changes needed to support transformation. The other reason is that big companies always feel the threat from smaller competitors with disruptive technologies and are eager to adopt technologies like AI to create a competitive advantage for them. The last reason is that they are the most affected by the loss of skilled manufacturing employees through retirement or resignations and they need to compensate for this loss with technologies like AI.
In engineering and design, quite a few of respondents highlight problems with data quality. In factory operations, again a lot of them point to weaknesses in data governance. The manufacturing industry generates enormous quantities of data, and research has shown manufacturers see growth in data volumes from their operations outstripping other industries.
In spite of the high investments in AI, the bigger companies are not able to gain immediate value from their AI models because as mentioned earlier, the data is simply not suitable for the AI models and hence there is a need for robust data management strategies like those offered by HiveMQ before the data can become suitable. This needs additional investments which companies, especially the bigger ones, have started to realize. We see this a lot with the number of inquiries from large organizations in various manufacturing industries including Food & Beverage, Pharmaceuticals, Automotive, and others around UNS and how they can get organized around that with data governance and security best practices.
Manufacturers further along in deploying AI use cases in production feel this problem especially keenly. A fourth of the respondents say the data quality is suitable for factory and production use cases. One of the production challenges is the limited interoperability between manufacturers’ OT and IT systems. OT, such as programmable logic controllers (PLCs) and supervisory control and data acquisition (SCADA) systems hold large volumes of machine data that AI models would benefit from.
Fragmentation of data must be addressed for AI to scale. Most manufacturers find some modernization of data architecture, infrastructure, and processes is needed to support AI, along with other technology and business priorities. A modernization strategy that improves interoperability of data systems between engineering and design and the factory, and between operational technology (OT) and information technology (IT), is a sound priority.
One of the ways to overcome this challenge in the production use case is by using an Edge gateway like the HiveMQ Edge (Fig. 3). This can help by interacting with PLCs, SCADA systems, sensors and other OT assets that have their own proprietary protocols, converting the data to a common format, transforming, normalizing, conceptualizing and enabling consolidating the data along with a network of other brokers to make it suitable for AI applications.
Fig 3: Typical Edge to Enterprise data architecture to enable AI applications
Creating the Data Foundations
As it relates to developing AI capabilities, manufacturing executives surveyed leave no doubts about where their chief data challenges lie (Fig. 4). More than half (57%) of all respondents name data quality as a top challenge. Almost as many (54%) cite the need to improve data integration. A third major imperative (cited by 47%) is improving data governance. These are closely interrelated challenges. The ability to meet any one of these hinges on success in addressing all of them.
Fig 4: Which of these present your organization’s biggest data challenges when it comes to AI?
Poor data quality results from a variety of factors. Errors in data entry, missing data points, inoperative sensors in plant equipment, and siloed data trapped in legacy systems are just some of the more common ones. Siloes, in turn, are a manifestation of inadequate data integration and are a significant impediment to scaling AI use cases.
As mentioned in the above sections, IIoT data management solutions like HiveMQ enable overcoming many of these data challenges through a suite of products and services.
Pressure to Profit from AI
Given the sizable increases in AI spending planned by manufacturers, the pressure will be on executives to demonstrate return on investment. The most common engineering/design stage benefits involve greater speed, improved efficiencies, and reduced error/failure rates. The most common production benefits include better product or process innovation, safety, and reduced carbon footprint.
IIoT Data Management Enables AI
As has been covered in this blog post, there are several concerns that manufacturing companies have, especially around data quality, governance, silos, and scalability, that are preventing them from fully adopting AI use cases. The good news is that several proven IIoT data management systems like HiveMQ can enable them to overcome some of these challenges. However, there are other things that need to align with tools, like people (especially leadership strategies and processes to enable a data-driven culture in organizations to reap the maximum benefits). Download the HiveMQ software and try us for free. Additionally, book a demo if you want to learn more.
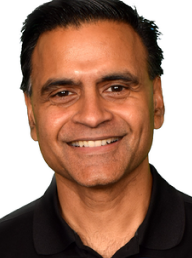
Ravi Subramanyan
Ravi Subramanyan, Director of Industry Solutions, Manufacturing at HiveMQ, has extensive experience delivering high-quality products and services that have generated revenues and cost savings of over $10B for companies such as Motorola, GE, Bosch, and Weir. Ravi has successfully launched products, established branding, and created product advertisements and marketing campaigns for global and regional business teams.