Importance of Data Governance and Integrity in Industrial IoT Use Cases
Data governance and integrity are an important part of Industrial IoT (IIoT) use cases, especially for Artificial Intelligence (AI), to ensure that data is accurate, consistent, and secure. Data governance establishes policies and procedures for how data is collected, stored, secured, and used, including how the system will comply with data security and privacy laws. Data integrity ensures the accuracy, completeness, consistency, and validity of data.
According to a Deloitte survey, manufacturing generates more data than any other industry (fig 1).
Fig 1: Annual data created by industry (in petabytes)
Given the amount of data in manufacturing, data governance and integrity becomes even more important. For organizations to adopt AI widely, there must be some way to manage the data and make sure it can be trusted to build the data models needed for AI.
Data governance and data integrity are critical to a manufacturer’s ability to monetize data effectively. They directly impact the accuracy, consistency, and reliability of data, which are essential for deriving actionable insights, making informed decisions, and ultimately driving profitability. Poor data governance and integrity can lead to inaccurate analysis, flawed decision-making, and missed opportunities for monetization. Here’s how these concepts influence data monetization and the strategies manufacturers should implement to maximize data value.
Impact of Data Governance and Data Integrity on IIoT Use cases
Following are some of the consequences and benefits of data governance and integrity that should be considered:
Trust in Data-Driven Decisions
Accuracy and Consistency
Data integrity ensures that the data used in decision-making is accurate, consistent, and free from errors. Without reliable data, the insights derived can be misleading, leading to poor decisions that could harm operations and profitability.
Data Quality
Data governance frameworks define standards and processes for maintaining high data quality, which is crucial for ensuring that the data is fit for its intended purposes, whether for internal decision-making or external monetization.
Regulatory Compliance and Risk Management
Compliance with Regulations
Manufacturers must adhere to various data-related regulations (e.g., GDPR, CCPA). Strong data governance ensures that data handling practices are compliant, reducing the risk of legal penalties and protecting the company’s reputation. A SOC 2 examination is a report on controls at a service organization relevant to security, availability, processing integrity, confidentiality, or privacy. SOC 2 Type 1 compliance evaluates an organization's cybersecurity controls at a single point in time. A SOC 2 Type 2 report is a third-party audit that assesses a company's internal controls and how well they protect customer data over a period of time. Manufacturers in service organizations are expected to have SOC 2 compliance on their data.
Risk Mitigation
Effective governance and data integrity help mitigate risks associated with data breaches, inaccurate reporting, and financial losses, which could undermine efforts to monetize data.
Efficient Data Monetization
Data as an Asset
High-quality data is a valuable asset that can be monetized in various ways, such as selling data, developing data-driven products, or optimizing operations. Data governance ensures that data is well-organized, accessible, and ready for monetization opportunities.
Enhanced Customer Value
Reliable data enables manufacturers to offer personalized products and services, improve customer experiences, and develop new revenue streams. Data integrity ensures that these offerings are based on accurate customer insights.
Internal and External Stakeholder Confidence
Building Trust with Partners
Reliable data builds trust with external partners and customers who may rely on the data for their own operations or decision-making. Poor data governance can lead to inconsistencies that erode this trust.
Internal Alignment
Strong data governance ensures that all departments within a manufacturing company have access to the same accurate data, enabling aligned decision-making and strategy execution across the organization.
Strategies to Ensure Data Accuracy, Consistency, and Reliability
Establish a Robust Data Governance Framework
Define Clear Policies and Standards
Industrial companies must develop and implement clear data governance policies that outline data ownership, data quality standards, and data management processes. They must ensure that these policies are aligned with regulatory requirements and industry best practices.
Data Stewardship Roles
Industrial companies must appoint data stewards or data governance officers responsible for overseeing data management practices, ensuring compliance with governance policies, and maintaining data quality across the organization.
Implement Data Quality Management Processes
Data Validation and Cleansing
Industrial companies must regularly validate and cleanse data to remove inaccuracies, duplicates, and inconsistencies. They must implement automated tools to perform real-time data quality checks and flag any issues for immediate resolution.
IoT data management providers like HiveMQ offer solutions such as Data Hub, an integrated policy and data transformation engine that automatically validates, enforces, and manipulates data in motion to ensure data integrity, quality and governance across the deployment.
Master Data Management (MDM)
Industrial companies should establish a master data management system to create a single, accurate source of truth for key data entities (e.g., customers, products, suppliers). MDM helps ensure consistency across all systems and processes.
One way to achieve that in the industrial world is by bridging the data from the Operational Technology (OT) systems and the Information Technology (IT) systems using a data framework called Unified Namespace (UNS), which provides a single source of truth for all of the organization's business data. The HiveMQ MQTT platform offers a very powerful UNS framework.
Leverage Technology for Data Governance and Integrity
Data Governance Platforms
Industrial users can utilize data governance tools like HiveMQ’s Data Hub, that provide centralized control over data policies, data lineage tracking, and access management. These tools help ensure that data is managed consistently across the organization.
Automated Data Quality Tools
Industrial users can also implement automated data quality tools that can continuously monitor data for accuracy, completeness, and consistency. These tools can identify and correct data issues in real-time, maintaining data integrity.
Promote a Data-Driven Culture
Employee Training and Awareness
Industrial companies must educate employees on the importance of data governance and integrity, and provide training on best practices for data management. They should encourage a culture where data quality is everyone’s responsibility.
Cross-Department Collaboration
Industrial companies must foster collaboration between IT, OT, and business units to ensure that data governance practices are understood and adhered to across the organization. This helps align data management with business goals. One way to foster the collaboration is by ensuring that a UNS is created using an enterprise data broker like HiveMQ which ensures that the data is consolidated in one location, connecting all the systems.
Ensure Data Security and Compliance
Data Access Controls
Industrial companies must implement strict access controls to ensure that only authorized personnel can access sensitive data. They should regularly audit data access logs to detect and prevent unauthorized access. Again a UNS created using the HiveMQ platform can help implement those strict access control policies and provide audit logs either within the tool or through external tools like Grafana.
Compliance Monitoring
Industrial companies should continuously monitor data handling practices to ensure compliance with relevant regulations. This includes implementing data encryption, anonymization, and other security measures to protect sensitive data. HiveMQ provides advanced security features which includes data encryption. HiveMQ is committed to using the highest information security and risk management standards to protect and handle customer data with the optimum level of trust and compliance. HiveMQ provides GDPR, SOC 2 type 1/type 2, ISO/IEC 27001 certification and CSA STAR registry for Cloud data.
Continuous Improvement and Auditing
Regular Audits and Reviews
Industrial companies should conduct regular audits of data governance practices and data integrity to identify areas for improvement. They should use these audits to refine data management processes and ensure ongoing compliance with governance standards.
Feedback Loops
Industrial companies must establish feedback loops where data users can report data quality issues or suggest improvements. They should use this feedback to continuously improve data governance practices and maintain high data quality.
Conclusion
In the context of IIoT, effective data governance and data integrity are indispensable for ensuring that the massive amounts of Industrial data generated are accurate, secure, and actionable. Strong governance frameworks enhance the value of IIoT data by improving decision-making, operational efficiency, regulatory compliance, and system scalability. As IIoT ecosystems continue to evolve and expand, the role of data governance will only become more important in ensuring the long-term success and reliability of industrial operations.
The HiveMQ MQTT platform is built for effective data management and can help with both data governance and integrity. Download HiveMQ and try us for free.
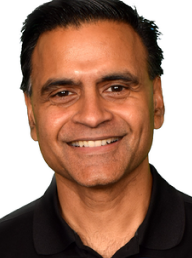
Ravi Subramanyan
Ravi Subramanyan, Director of Industry Solutions, Manufacturing at HiveMQ, has extensive experience delivering high-quality products and services that have generated revenues and cost savings of over $10B for companies such as Motorola, GE, Bosch, and Weir. Ravi has successfully launched products, established branding, and created product advertisements and marketing campaigns for global and regional business teams.