Why is Overall Equipment Effectiveness (OEE) an Important KPI for Industrial Processes?
Manufacturers strive to ship quality products on time, while controlling costs. This can be very complicated with factors like labor shortages and machine downtime preventing companies from achieving maximum productivity. While manufacturers continue to work to mitigate these challenges, there isn’t always a straightforward process to follow when tracking and improving operations. Overall Equipment Efficiency (OEE) can help.
OEE is a Key Performance Indicator (KPI) that helps manufacturers determine the machine potential in their factory or production lines.
How is OEE Calculated?
The most common and simplest way to calculate OEE is:
OEE = Availability x Performance x Quality
Availability refers to the ratio of the actual production time (Fully Productive Time) to the Planned Production Time. Quality refers to the quality of products and the number of defects. Performance focuses on production process speed and how long equipment maintains that pace. While there may be other methods, this calculation is the preferred way to track OEE, yielding a score to provide insights and an accurate picture of how effectively the manufacturing process is running. It makes it easy to track improvements in that process over time. For the purposes of this article OEE will refer to this preferred calculation method. Manufacturing operations worldwide use OEE metrics as part of their KPIs to better understand their production systems. They can use that KPI to identify, monitor, and reduce the amount of production loss they experience over a given period, directly impacting the bottom line.
Because OEE is such an important KPI, it’s equally important that manufacturers do everything they can to improve it, using data to take corrective actions to reduce or limit production losses.
OEE and Effective Production
There is a tight correlation between effective production and OEE which is described below in Figure 1. Perfect production is defined as an OEE score of 100 percent, which means the manufacturer is only producing high-quality components as rapidly as possible with no downtime. For discrete manufacturers, an OEE score of 85 percent is considered world-class and is a long-term objective. A 60 percent OEE score is common for discrete manufacturers, indicating that there is still a lot of opportunity for development. An OEE score of 40 percent is considered poor, but it’s not unusual for manufacturers that are just getting started with performance tracking and improvement. In most situations, a poor score may be readily improved by OEE tracking and taking corrective actions.
Figure 1: OEE Benchmarking
What are the Key Benefits of OEE?
Here are some of the key benefits that Manufacturers can expect by effectively tracking and improving OEE:
Return on Investment (ROI): Companies heavily invest in machinery, and it is crucial to receive the maximum ROI. Tracking OEE as a KPI may have a significant impact on the company’s bottom line by allowing them to optimize, thus producing more products on the same equipment in the same time period.
Maximize Workforce Productivity: OEE can help companies figure out why they experience operator downtimes, provide productivity statistics, and highlight delayed changeovers or setup times. This type of information helps in resource allocation, identifying areas of surplus capacity and determining where additional personnel are required.
Easily Visualize Performance: OEE focuses on visibility, allowing companies to visualize production difficulties rather than relying on their best estimate. Everyone can see what is working and where improvements are required by combining the major drivers of productivity losses into a single percentage.
Challenges Collecting Data for OEE
Continuous measurement of OEE requires the ability to bring together different data sources such as ERP, MES, and IoT data from machines on the shop floor, as well as quality data, test measurements and inspection data in different timeframes. The collection and tracking of OEE data is even more important in regulated industries like Pharma where it can provide product traceable and consistent documentation.
Here are some of the challenges with the legacy data collection methods:
Human errors and delays: When workers manually track important, particularly detailed data, they are under enormous pressure to get it right. Obviously, this can create room for error, especially as workers take measurements dozens of times in a single shift. Organizations also may have to train staff to fully understand and accurately collect and transcribe data. Manual data collection can also take more time.
Connectivity and bandwidth challenges: Factory networks are typically constrained by lack of connectivity and low bandwidth. Also machines speak different languages. Due to this manufacturers find it difficult to get real time and reliable data to be able to track OEE.
Cybersecurity concerns: Hackers can access a manufacturer’s data through older systems that don’t always come with proper digital security measures.
Lack of scalability: When businesses grow and diversify, they may need to expand their quality control processes. Data collection systems must be scalable to accommodate this. Lack of scalability can result in inaccuracies, delayed communication and can impact quality.
Incomplete analysis: Sampling strategies are where a small sample of data is used as a representation of the data quality from the entire system. They may impair predictions and the ability to identify changes in production tolerances. Some industries, such as Pharma, won’t even allow it as a way to monitor quality.
Supporting different data types: Machine data comes in different intervals and different volumes and legacy data collection methods don’t account for. This can result in data packets being lost and inaccuracies in the OEE calculation.
Digital Transformation, Industry 4.0 and Industrial IoT to Manage OEE
Many manufacturers are looking to Digitally Transform themselves and adopt Industry 4.0 or Industrial IoT (IIoT) solutions to better track, analyze and improve OEE. The legacy methods make it hard to identify losses that contribute to low machine productivity as they are difficult to identify, record, and analyze. Realizing Industry 4.0 and IIoT does not have to necessitate a major infrastructure overhaul that costs a lot of money. Advanced IIoT solutions that combine hardware and software solutions can provide turnkey solutions for data acquisition, data aggregation, visualization and central management to support OEE use cases effectively and in a timely manner.
Data Acquisition and Aggregation
OEE Data from manufacturing machines, processes, and applications are acquired, stored and aggregated via key ingestion technologies. On the operational technology (OT) side, data is stored with controllers, PLCs, gateways, and edge devices, and on the IT side like ERP, MES and other data, it is stored in a datacenter or enterprise cloud. Data storage technology enables the long-term storage of digitized data captured from advanced sensors and systems. This data-rich environment that converges IT and OT data enables advanced OEE tracking and analytics use cases as shown in Figure 2.
Figure 2: OEE Data movement using IIoT systems
A Data Broker is a key technology enabler for IIoT to enable this data convergence. A data broker is an intermediary entity that enables OT and IT client systems to communicate with each other. Using an underlying standard such as MQTT Protocol, a data broker supports the ability to connect multiple clients publishing data and multiple clients that are subscribed to receive the data such as enterprise applications. The clients communicating with the broker can abstract the underlying protocol that the machines/processes use to communicate. The broker works well in low bandwidth environments with unreliable communication mechanisms due to the underlying publish/subscribe method, where machines/processes don’t need to keep polling to get the OEE data.
OEE Combined with IIoT is Powerful
As discussed in this blog, OEE is a powerful way to measure production efficiency. A simple OEE score can be easily interpreted, tracked over time, and acted upon to provide many benefits to manufacturers. There are lots of challenges in OEE data collection, and data brokers can play a very important part in the process. In the second part of this article, we will go over MQTT Sparkplug and how it can enable seamless OEE data acquisition and aggregation. Read our blog to explore 5 ways MQTT Sparkplug helps improve OEE in industrial processes.
Contact HiveMQ to enable OEE improvement and join 150+ customers that have trusted us with their data needs.
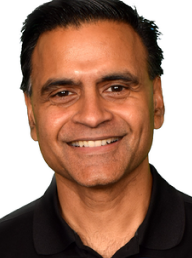
Ravi Subramanyan
Ravi Subramanyan, Director of Industry Solutions, Manufacturing at HiveMQ, has extensive experience delivering high-quality products and services that have generated revenues and cost savings of over $10B for companies such as Motorola, GE, Bosch, and Weir. Ravi has successfully launched products, established branding, and created product advertisements and marketing campaigns for global and regional business teams.