Data Mastery Enabling Key Manufacturing 4.0 Experiences
The Manufacturing 4.0 journey is marked by a growing dependence on industrial data as the driving force behind operational excellence and business growth. The findings from a recent survey on data mastery in manufacturing by the Manufacturing Leadership Council (MLC) underscore data’s pivotal role in shaping the future trajectory of manufacturing, highlighting both the opportunities and challenges that lie ahead. While advancements in technology promise a wealth of new possibilities, including real-time data collection, predictive analytics, and automation, there remains a significant gap between available data sources and their optimal utilization. The prevalence of manual data entry, the disparity between traditional and emerging technologies, and the ongoing struggle with standardized data formats, data quality and data inconsistency all point towards a need for concerted efforts to harness manufacturing data’s full potential.
Looking forward to the year 2030, manufacturing data is poised for significant transformation, with an increasing emphasis on proactive decision-making, predictive analytics and leveraging data for improved operational efficiencies. As Manufacturing 4.0 continues to evolve, the role of AI and other digital technologies will become more established, and perhaps reshape processes, roles, and manufacturing itself.
As manufacturers proceed on their data journeys and look forward to 2030, solving the organizational issues evidenced in this survey will be central to their ability to maximize the value from the data they are collecting about their operations.
The first step in this process is understanding the inconsistencies and the tension points arising from being a data-driven business. Data mastery can be achieved when that understanding leads to workable solutions successfully applied. Only then will the promise of using data to both improve operations and find new and better ways of running manufacturing plants be realized.
Data Governance and Organization
From a data ownership perspective, what was once the sole responsibility of IT — data ownership at manufacturers — is moving to shared responsibility with IT/OT while the responsibility for good quality data is moving to the business and senior leadership teams according to the survey.
Data management includes traditional elements, such as data security and privacy, along with data quality and governance. Data security and privacy are considered essential business requirements, and survey respondents validate this. The continued rise of AI and analytics solutions is driving an increased focus on data governance and data quality, but companies are just starting to apply the same rigor here as they do on security and privacy.
When asked the question about policies on data quality Fig. 1), 28% of the manufacturers responded that they have a formal policy on manufacturing data quality. Most of these companies manage the data through individual silos and systems. Developing a formal company-wide policy for data quality is important for manufacturers to realize the full potential of integrated datasets supporting activities such as AI, digital threads, and digital twins.
Figure 1: Does your company have a formal policy on data quality? (select one)
More than 60% of respondents have a corporate-wide plan, strategy, or guidelines for data management (Fig. 2), but interestingly only 15% follow this entirely. The reason for this could be how organizational data is siloed, difficult to combine as it is in different formats, not trustable as it has a lot of quality issues. This is, however, changing as companies realize that to truly leverage AI and other advanced analytics, they need to start with data engineering and do a better job of collecting, organizing, cleansing, normalizing, accessing, and utilizing data. And, as the survey shows, it is not enough to just have policies in place; companies need to have the processes, technology, and skills in place to ensure these policies are being followed.
Figure 2: Does your company have a corporate-wide governance plan, strategy or formal guidelines for how data is collected, organized, accessed and utilized across the enterprise, including manufacturing operations? (select one)
The Manufacturing Leadership team plays one of the most vital roles in digital transformation, including data projects. Most survey respondents agreed on this when 58% of them mentioned that leadership had the primary data governance responsibility. In spite of this, one of the other reasons why these data management policies are not implemented is because in most cases, there was no direct connection between data activities and annual incentives, KPIs, or business imperatives for their leadership team. Only 22% of the respondents said that there was a connection in their organization (Fig. 3).
Figure 3: Is data collection/management/analysis included in annual incentives, KPIs or business imperatives for company executives/leadership? (select one)
Based on the insights above, one of the ways to implement an effective data management strategy is by using an Internet of Things (IoT) data solution like HiveMQ which offers a suite of solutions enabling an enterprise-grade data management solution.
One of the ways HiveMQ is able to support our customers on this journey is by implementing a data framework named Unified Namespace (UNS) which serves as the single source of truth for all contextualized data and information of the manufacturing business. At the core of UNS is the Enterprise data broker offering a highly scalable, reliable, and flexible data solution. The other key aspect of this is the Data Hub, which provides an integrated policy and data transformation engine that validates, enforces, and manipulates data in motion to ensure data integrity, quality and governance across the deployment.
Manufacturing Data
As the amount of manufacturing data compounds, manufacturers are grappling with the challenges of collecting, analyzing, and leveraging the abundance of data now at their fingertips. The confluence of traditional and modern technologies and practices, alongside divergent data standards, are hurdles to fully harness the potential of the data being generated. It is important to overcome these obstacles now because as survey respondents have suggested, data will be even more vital to manufacturing’s future.
Manufacturers have to deal with many systems on the factory floor that houses the data, and most of the current ways of analyzing that data involve manual practices as agreed by 70% of the survey respondents (Fig. 4). Traditional systems like PLCs, quality systems, and ERP remain prevalent as data sources, newer technologies, including vision systems, AI, edge computing, and digital twins lag behind. While there is a recognition of these technologies' potential, barriers continue to impede their widespread adoption and integration within manufacturing processes.
Figure 4: What are the sources of your manufacturing data today? (select all that apply)
As use of these newer technologies becomes more prevalent, the number of data sources will continue to expand in manufacturing settings. As it does, widespread manual data entry will become a less sustainable option. Some 44% of manufacturing leaders have seen at least a doubling of the amount of data they are collecting in their organization today compared to two years ago. Half of the leaders surveyed expect the data they collect to at least triple by 2030. With all this data, analysis and generating insights becomes a key responsibility of the continuous improvement team as the data volumes keep increasing. 68% of the respondents agreed with this notion, with factory supervisors and OT teams being close second and third options (Fig. 5).
Figure 5: Organizationally, which groups have primary responsibility for analyzing and generating insights from your manufacturing data? (select all that apply)
To be able to handle such volumes of data and to be able to talk to multiple systems to consolidate the data, an enterprise-grade data solution that offers high scalability and reliability like HiveMQ is needed. One of the powerful products offered is HiveMQ Edge which enables interoperability between the various OT and the various IT systems by translating diverse machine protocols into the standardized formats, modernizing IoT infrastructure, and making seamless edge-to-cloud integration a reality. The other product offering that complements this are HiveMQ Extensions, which provide seamless data integration with streaming, analytics and database platforms; offer robust data security; and support comprehensive monitoring to enhance application performance.
Business Impact
Measuring manufacturing data value is not the same as using data to recognize the value in Manufacturing 4.0 projects. Investments in how data is collected, organized, accessed, and utilized need to be balanced with the value that is generated by that data through improved analytics, insights, and decision making. By treating data as an asset with financial (or other) value, companies can apply lean principles (e.g., minimize waste, drive efficiency, think of value streams) to ensure data is not being wasted.
What most manufacturers are finding is that only 25% of them have high confidence that the data they are collecting will indeed generate the value (Fig. 6).
Figure 6: How would you rank your company’s ability to collect the right data the business needs from your manufacturing operations? (select one)
The other way to look at the chart is that only 17% have low confidence in the data’s ability to generate value. Diving into the actual value that the data can generate, it is no surprise that respondents picked the usual suspects like quality, cost reduction, efficiency, and speed as the areas which would have the most impact (Fig. 7).
Figure 7: How has the increase in manufacturing data helped you to improve your manufacturing organization since 2020, or whenever you started your digital journey, to now? (whichever is earlier)
As mentioned in the above sections, the best way to increase manufacturer confidence is by providing power data integrations, improving data quality, consolidating the data from various sources, and enabling advanced applications like AI, Digital Twins, and other advanced analytics to be effective by using IoT data solutions like HiveMQ. This point was also made by the survey respondents to the question of how easy it is to share the data within the organization, where only 41% responded that the data is easily shareable. This indicates that silos still exist and will need to be broken down to reach the desired future state (Fig. 8).
Figure 8: Is data routinely shared across any or all of the following parts of the organization? (select all that apply)
Future Trends
Data sharing across silos is of concern today as mentioned above. The survey shows that integration between systems is the number-one concern manufacturers see preventing them from achieving their goals. On the positive side, there is very little concern about the ROI of data-driven decision-making, so it is a matter of improved data governance, management, and skills using data solutions like HiveMQ.
Figure 9: What are the most important challenges or obstacles hindering your organization from making more data-driven decisions? (select top three)
Based on all the responses, it's clear that data will be a crucial aspect of future competitiveness, which is clearly a powerful statement about the future of manufacturing data. An overwhelming majority (86%) said that data will be essential, which again highlights the need to improve data collection, standardization, normalization, analysis, and usage.
Conclusion
The results of this MLC data mastery in manufacturing survey show that the manufacturing industry is still in the grips of too much data but too little useful data. It also shows that manufacturers are making progress on improving data management which includes governance and quality. Data volumes are growing too fast, and new technologies, such as generative AI tools, are coming to market too quickly for that to happen. Seizing the data opportunity requires strategic thought, persistence and adaptability. Manufacturing companies that achieve data mastery will know it when they do. They will innovate faster and more frequently, be more efficient and agile, and become more competitive in their markets. When that happens, all the hay will be woven into gold.
IoT solutions like HiveMQ, and others can help manufacturers achieve data mastery which then helps enable key Manufacturing 4.0 experience. Download HiveMQ software and try us for free.
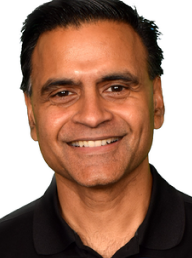
Ravi Subramanyan
Ravi Subramanyan, Director of Industry Solutions, Manufacturing at HiveMQ, has extensive experience delivering high-quality products and services that have generated revenues and cost savings of over $10B for companies such as Motorola, GE, Bosch, and Weir. Ravi has successfully launched products, established branding, and created product advertisements and marketing campaigns for global and regional business teams.