Bridging the IT-OT Divide to Enable Industrial Use Cases
Over the past few years, data transformation has delivered significant value to industrial users across various domains. In quality control and assurance, it has enhanced product inspections and optimized processes. In supply chain management, it has enabled real-time visibility and more accurate demand forecasting. In energy management, data transformation has improved efficiency and advanced sustainability efforts, such as reducing carbon footprints. For product mass customization, it has facilitated personalized production, strengthened customer feedback loops, and enabled lot size 1 manufacturing. In the realm of cybersecurity, it has bolstered data protection and improved compliance and risk management. These are just a few examples, with many other areas also benefiting from data transformation.
One of the main value drivers is the significant improvements being made in the fields of industrial data collection and analysis.
Trends in Industrial Data Collection and Analysis
Some of the significant trends in industrial data collection and analysis are:
Industrial Internet of Things (IIoT)
Widespread Sensor Deployment
The proliferation of cheap and powerful IoT sensors in industrial environments has led to the collection of massive amounts of real-time data from machines, equipment, and processes. This data is crucial for monitoring performance, predicting failures, and optimizing operations.
Connected Infrastructure
IIoT enables the creation of fully connected infrastructure-like factories, operations and others where data flows seamlessly across all levels of the organization. This connectivity supports real-time decision-making and greater agility in responding to production challenges.
Edge Computing
Real-Time Data Processing
Edge computing allows data to be processed close to the source of generation, reducing latency and enabling real-time analytics. This is particularly important in industrial use cases, where immediate insights are needed to optimize production processes and ensure quality.
Reduced Data Transfer Costs
By processing data locally, and only sending relevant data, edge computing reduces the need to transfer large volumes of data to central cloud systems, lowering costs, improving data security and increasing data relevancy.
Artificial Intelligence (AI) and Machine Learning (ML)
Predictive Analytics
AI and ML are increasingly being used to analyze historical and real-time data to predict equipment failures, optimize production schedules, and improve supply chain efficiency. These predictive capabilities are essential for minimizing downtime and reducing operational costs.
Quality Control Automation
AI-driven image recognition and anomaly detection are revolutionizing quality control by identifying defects and inconsistencies in real-time, leading to higher product quality and reduced waste.
Big Data and Advanced Analytics
Integration of Diverse Data Sources
Industrial users are collecting and analyzing data from a wide range of sources, including production systems, supply chains, customer feedback, and market trends. This holistic approach to data integration enables more comprehensive insights and better decision-making.
Advanced Simulation and Modeling
Big data analytics in conjunction with digital twins is being used to create detailed simulations of industrial processes, allowing companies to optimize production parameters, reduce cycle times, and innovate more rapidly.
Cloud Computing and Data Platforms
Scalable Data Storage and Processing
Cloud computing provides the scalability needed to store and process vast amounts of industrial data. Industrial users are leveraging cloud-based platforms for data analytics, collaboration, and innovation.
Integration of AI and IoT
Cloud platforms are increasingly integrating AI and IoT capabilities, allowing industrial users to develop and deploy advanced data-driven applications more quickly and at a lower cost.
The OT IT Divide Impeding Data Collection Adoption and Analysis
In spite of these significant technological advancements that have happened in recent times, adoption of these trends have been slow due to the significant divide between companies' Information Technology (IT) and Operations Technology (OT) teams and initiatives. Integrating OT with IT to create data analysis systems in industrial companies is a powerful way to enhance operational efficiency, but it comes with several key challenges. These challenges often stem from the differences in the objectives, systems, and cultures of OT and IT departments. However, with the right strategies, companies can bridge the gap between these two areas to ensure seamless collaboration and effective data-driven decision-making. Here are the key challenges and strategies for overcoming them:
Cultural Differences Between OT and IT
Different Priorities and Objectives
OT is primarily focused on maintaining uptime, reliability, and safety in industrial operations, while IT is more concerned with data management, cybersecurity, and network stability. This can lead to differing priorities and resistance to change.
Organizational Silos
OT and IT departments often operate in silos, with limited communication and collaboration. This separation can hinder the integration of systems and data.
Legacy Systems and Compatibility Issues
Outdated OT Systems
Many OT systems in manufacturing are legacy systems that were not designed with modern IT integration in mind. These systems may use proprietary protocols, making integration with IT systems challenging.
Lack of Interoperability
OT systems often use different communication protocols and standards compared to IT systems, leading to compatibility issues when trying to integrate them.
Cybersecurity Concerns
Increased Attack Surface
Integrating OT with IT can expose critical industrial systems to cybersecurity threats, as these systems become more connected to broader networks. OT systems are often less secure and more vulnerable to attacks.
Differing Security Approaches
IT and OT have traditionally had different approaches to security. IT focuses on protecting data confidentiality and integrity, while OT prioritizes system availability and safety. This can lead to conflicts in security strategies.
Data Integration and Management
Data Silos
OT and IT systems often generate and store data in separate silos, making it difficult to integrate and analyze data across the entire organization.
Data Quality and Consistency
The quality, format, and frequency of data generated by OT systems may vary significantly, making it challenging to ensure consistent and accurate data for analysis.
Skill Gaps and Workforce Resistance
Lack of Cross-Disciplinary Skills
The skills required to manage and integrate OT and IT systems are often different, leading to a gap in expertise. Few employees have a deep understanding of both areas.
Resistance to Change
Employees in both OT and IT departments may resist the integration due to concerns about job security, increased workload, or the need to learn new technologies.
Strategies to Bridge the OT IT Divide
Foster Cross-Departmental Collaboration
Creating Cross-Functional Teams
Establishing cross-functional teams that include members from both OT and IT departments enables teams to work together to develop integration strategies, address challenges, and ensure alignment with organizational goals.
Promoting Communication and Understanding
Encouraging regular communication between OT and IT teams helps build mutual understanding of each other’s priorities, challenges, and perspectives. This can help reduce cultural barriers and foster collaboration.
Implementing Standardized Protocols and Middleware
Adopting Industry Standards
Using standardized communication protocols such as OPC UA for machine to machine communication and MQTT for Machine to Enterprise or Machine to Cloud communications can facilitate interoperability between OT and IT systems. This can help reduce compatibility issues.
Using Middleware Solutions
Implement middleware that can bridge the gap between OT and IT systems by translating data formats, managing communication, and ensuring that data flows smoothly between different systems.
Enhancing Cybersecurity Measures
Implementing a Unified Security Framework
Developing a comprehensive cybersecurity strategy that addresses the unique needs of both OT and IT environments. This includes segmenting networks, using firewalls, and deploying intrusion detection systems (IDS) tailored for industrial environments.
Continuous Monitoring and Threat Detection
Deploying continuous monitoring tools that can detect and respond to security threats in real-time across both OT and IT systems. Regularly update and patch systems to address vulnerabilities.
Investing in Data Integration and Analytics Platforms
Unified Data Platform
Implementing a unified data platform that can collect, store, and analyze data from both OT and IT systems that enables support real-time data processing and that’s scalable to accommodate growing data volumes, variety and veracity helps bring the IT and OT teams together to solve common data goals and support digital transformation.
Data Governance Framework
Establishing a data governance framework that ensures data quality, consistency, and security helps the IT and OT teams achieve the common data goals. These goals could include setting data standards, managing metadata, and implementing data stewardship roles.
Data frameworks like Unified Namespace (UNS) provide a platform for the industrial companies to have a single source of truth for all business data and also helps establish the governance framework to achieve the common goals.
Developing Cross-Disciplinary Skills
Training Programs
Investing in training programs that help OT personnel gain IT skills and vice versa helps a lot with reducing the divide. This could include cybersecurity training for OT staff or OT process training for IT professionals.
Hiring and Developing Hybrid Roles
Recruiting and developing talent with expertise in both OT and IT, or creating hybrid roles that bridge the gap between the two domains is key. This can help ensure that integration projects are managed by individuals who understand the complexities of both environments.
Starting with Pilot Projects
Small-Scale Pilots
Starting with small-scale pilot projects that integrate specific OT and IT systems is important. These pilots should then be used to identify potential challenges, test integration strategies, and demonstrate the value of the integration to the broader organization.
Iterative Approach
Using an iterative approach to scaling up integration efforts, learning from each phase and gradually expanding the scope of the project based on successes and lessons learned is part of the best practices.
Change Management and Communication
Leadership Support
Ensuring that leadership is committed to the integration of OT and IT, and clearly communicates the strategic importance of this initiative to all stakeholders is crucial.
Addressing Resistance
Engaging with employees to address concerns and resistance to change is an important step. This could involve clear communication of the benefits, involvement in the planning process, and providing adequate support and resources for transition.
HiveMQ: Enabling the Best Practices in Data Collection, Consolidation and Management
HiveMQ empowers businesses to digitally transform with the most trusted MQTT platform. Designed to connect, communicate, and control IoT data under real-world stress, the HiveMQ MQTT Platform is the proven enterprise standard and powers Industrial use cases.
HiveMQ offers multiple products like the Enterprise MQTT Broker to consolidate industrial data at an enterprise level and offering the UNS single source of truth; the Edge Gateway to convert data from legacy industrial protocols to MQTT, bridge the data to the plant or enterprise level broker; Data broker which offers data policies that help transform, normalize, consolidate and contextualize at the edge, plant and enterprise levels and Extensions that enable data from the MQTT broker to be sent to IT databases, cloud applications, Historians and other IT systems. Download HiveMQ and try us for free today.
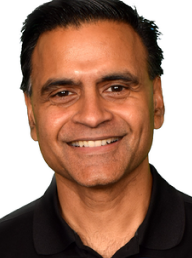
Ravi Subramanyan
Ravi Subramanyan, Director of Industry Solutions, Manufacturing at HiveMQ, has extensive experience delivering high-quality products and services that have generated revenues and cost savings of over $10B for companies such as Motorola, GE, Bosch, and Weir. Ravi has successfully launched products, established branding, and created product advertisements and marketing campaigns for global and regional business teams.